Good AI needs good data — and that’s a problem in government
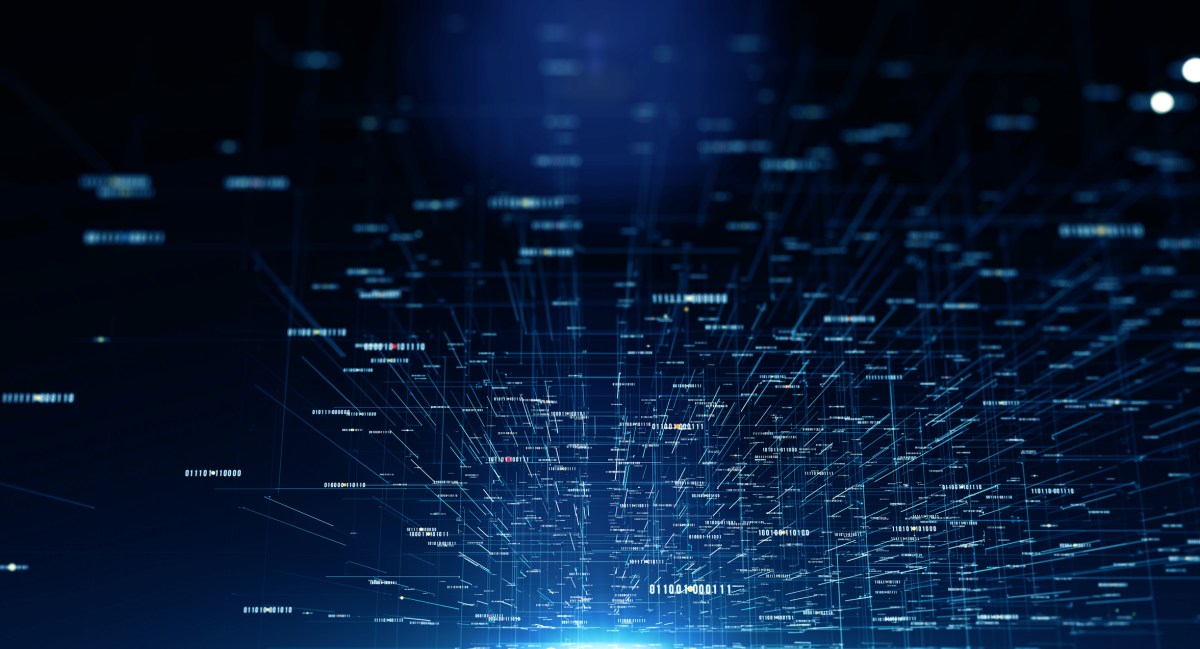
The uses for machine learning are growing at an unprecedented rate. Yet, as machine learning and its applications advance, the bottlenecks inhibiting its large-scale adoption are becoming clear. A lack of good, labeled data is an impediment to good AI.
Researchers need labeled data because machine learning first requires training a model. For example, if we want a model that predicts whether a consumer complaint is about cyber fraud or financial fraud, we would need to first label fraud complaints as either “cyber” or “financial.” We would then use the labeled data to train the model and eventually turn the model on real-time complaints.
At the moment, though, the difficulty of labelling data quickly and accurately means that barely any training data is available. Digital data is more than doubling every two years — and is projected to reach 44 trillion gigabytes by 2020, the International Data Corporation (IDC) reported in 2014. Yet 0.5 percent or less of these data is being analyzed, and only 3 percent has labels, according to the IDC.
Breaking Through the Training Data Bottleneck
Currently, most labeling is done by hand, a time-consuming task that the government has neither the time nor resources to do.
One solution is to use automated methods, such as Quickcode, a data-labelling tool created by DC-based startup Thresher. Using Quickcode, a data scientist spent 15 minutes to label 50,000 patient discharge summaries in order to train a model to predict readmission risk. Similar work by hand would have taken days to complete.
Without Quickcode, it’s likely that the labelling — and the resulting insights — would never have gotten done. “Discharge summaries contain an incredible amount of valuable health information” said Patrick Lam, Thresher’s lead data scientist, “But it’s often disregarded in models of patient outcomes, simply because it is too difficult and time-consuming to label them for use as a structured variable.”
Removing AI from Its Black Box
Labeled data, however, is only the half the story. In order to use it, data scientists and their partners in government need to trust that their data labelling programs are accurately categorizing their data.
Quickcode helps solve this problem by relying on a keyword system. Each time the user adds a keyword, Thresher rapidly iterates with the user and provides better recommendations. On each iteration, the data scientists can determine for themselves whether the labelling is going in the right direction. In tests against data that were individually hand-labeled, Quickcode matched the hand labels with 95 percent accuracy at a fraction of the time the hand-coding took.
“Thresher’s model is powerful because it takes the best of humans — detailed expertise, understanding of context and nuance — and the best of machines —the ability to process large amounts of data and detect patterns — to transparently label data orders of magnitude faster than would otherwise be possible,” said Becky Fair, Thresher’s CEO.
The U.S. government has vast troves of unstructured text data that, if labeled, could provide valuable insights for everything from healthcare to counter-terrorism. The challenge will be for companies — including Thresher — to give government the high-quality data it needs.
Shannon Hynds is customer success manager for Thresher. Thresher is a software company that builds machine learning tools to allow humans do what they do best: create sharp insights. Thresher was a graduate of the Dcode AI and Machine Learning Accelerator. Dcode is holding an IoT and Mobile Demo Day on April 5th, RSVP here: http://bit.ly/iotmobiledemoday