A new idea for AI regulation would give more authority to federal agencies
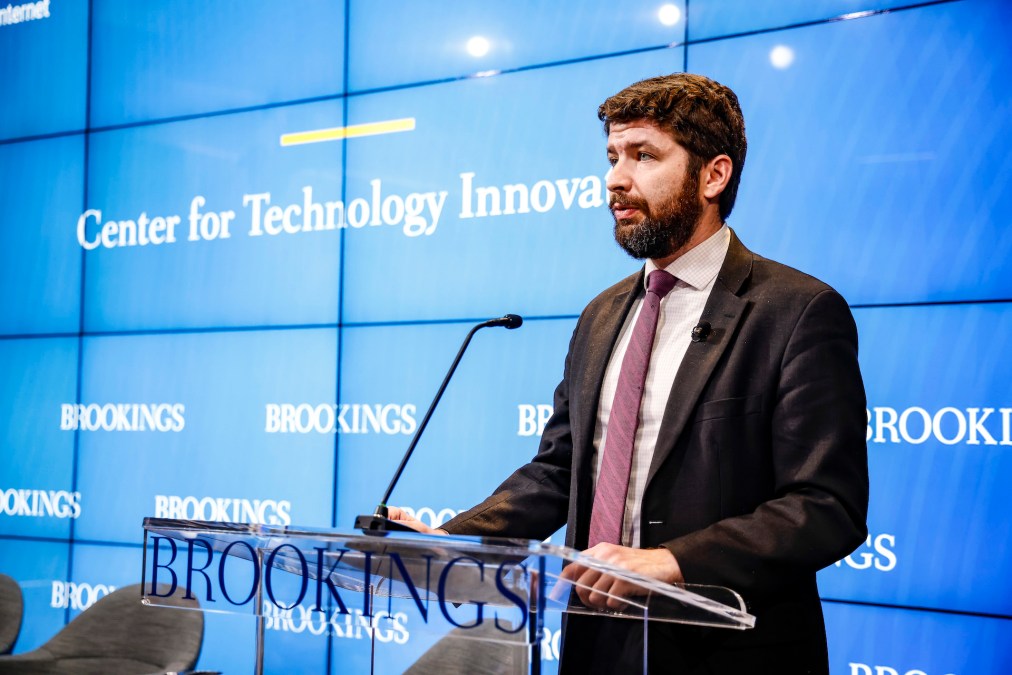
A new idea to regulate artificial intelligence has been designed to acknowledge the enormous challenge of overseeing a technology that’s already integrated into a range of systems that impact everything from a person’s job prospects to the price of a home.
The proposal, which was assembled by Brookings Institution fellow and AI policy expert Alex Engler and published late last month, is designed as an imperfect solution. The ideal approach to regulating the technology would involve a massive overhaul of civil rights laws for the age of AI, Engler notes. But that doesn’t appear politically feasible at the moment.
Engler’s concept focuses on governing algorithms based on their specific applications — and on expanding the power of regulatory agencies. He calls this approach the Critical Algorithmic Systems Classification, which would work, as he explained in his proposal, by granting these agencies authority to create rules around “especially impactful algorithms” and issue administrative subpoenas for algorithmic investigations.
The agencies that might be covered by the proposal include the Department of Education, the Equal Employment Opportunity Commission, and the Department of Health and Human Services, among plenty of others.
There are areas this regulatory method doesn’t cover, Engler told FedScoop, including the prospect of a private right of action and the existence of large language models. This policy strategy would also require federal agencies to significantly scale up their AI-focused staff. Still, Engler says there’s interest in the idea, particularly in advance of the upcoming Senate AI Insight Forums.
“The Schumer-ian process is attracting a lot of attention and an appetite for some new ideas,” Engler told FedScoop. “I do expect it to get seriously considered by some offices.”
In a recent interview, FedScoop spoke with Engler about what he’s trying to accomplish with the Critical Algorithmic Systems Classification, why it’s imperfect and why he believes it’s better than the alternatives.
Editor’s note: The transcript has been edited for clarity and length.
FedScoop: Can you start by explaining the problem you’re trying to solve?
Alex Engler: My general framing of the problem is that there are a whole bunch of important decisions made about people’s lives that used to be done by humans and are now primarily, predominantly, overwhelmingly done by algorithms. This includes decisions that dramatically affect people’s lives, especially in socioeconomic outcomes and their health, like, who gets the job and how much you earn at that job, the availability of loans, like mortgages, the valuation of the property that you own, whether or not you get into college and how much tuition costs, how much health care you get, and what your insurance will pay for.
That series of economic, socioeconomic, and health determinations all share a pretty big transition from primarily human-made decision-making to primarily algorithmic decision-making.
Our civil rights and consumer protections aimed at this set of problems were written 30, 40, 50 and 60 years ago, and no longer apply neatly and comprehensively to that challenge.
FS: It sounds like your proposal is an imperfect solution, given that there isn’t going to be, in the near term, a massive overhaul of several civil rights laws in order to deal with AI?
AE: The best policy solution is a detailed and incremental change to all of our civil rights laws to make sure that we’re accounting for the role of algorithms. But that is so incredibly labor intensive for legislators, for civil society advocates, and experts. And further, [it] would be very politically fraught to the point where a lot of groups you would expect to support that may not because of political risks. You could end up undermining the civil rights laws, potentially, or reducing their scope by opening them up to a giant debate.
FS: Can you explain to me the Critical Algorithmic Systems Classification tool? How does this work?
AE: The classic example here is AI hiring. We’ve had quite a bit of news coverage around potential discrimination [and] demonstrated discrimination in AI hiring systems.
With the CASC, in this case, the Equal Employment Opportunity Commission would have the investigative authority to go say: “Hey, we are worried about this, we’re gonna go collect data, and code and models and do interviews with the companies developing and deploying these systems.”
They would have that explicit authority with administrative subpoena access. And if they say, “Well, we think this rises to the scale of a critical algorithmic system that has risks and harms […] affecting lots of people, we’re going to create rules setting a minimum standard for its use.”
And that is what the CASC allows: If you find and demonstrate that an algorithm is doing that, you can set rules for its accuracy, its robustness, its nondiscrimination, how it informs users that it’s happening, how it lets users fix mistakes and data.
FS: Why is this better than creating a separate federal AI-focused agency, as some have suggested?
AE: I’m trying to solve a specific problem, and that problem is these socioeconomic determinations. We have had regulations and laws around these socioeconomic determinations for decades […] If you create just an AI regulator — and you give them these specific authorities to regulate this stuff — suddenly you have two different government bodies regulating the same process, depending on whether it’s done by a human or an algorithm. That’s not going to work very well.
Now, there are challenges. You do have to build staffing [and] make sure that agencies have the algorithmic expertise. They already have the domain expertise. They probably need more algorithmic expertise. But the government has to adapt to this. There’s no solution to any AI governance that doesn’t involve making sure there are career pathways and technical expertise in government.
An AI agency might also want to do other things that my solution doesn’t solve. And maybe it’s still worth considering. I don’t tackle just the existence of large AI models. I don’t tackle regulating online platforms — and we certainly need online platform governance in the United States. There are other things you can imagine a central AI body doing that would be useful.
FS: What does this mean for generative AI?
AE: It does apply to generative AI in the scenario where you use it for a high-impact socioeconomic determination. This whole approach is agnostic to the type of algorithm. If you use an algorithmic system, for any of these types of decisions, it can be regulated by an agency. The goal here is to avoid having a solution dictated by the type of algorithmic system.
We want this approach to work on algorithms that are 40 years old, like decision trees. We want it to work on the last ten years of deep learning. And we want it to work on whatever comes next.
It is truly agnostic to algorithms. One example is using computer vision to detect the condition of a house to value the house […] There’s no reason this approach wouldn’t apply to that scenario. To the extent that there are advanced AI systems being built into these processes, it totally solves that problem.