How NIH’s National Library of Medicine is testing AI to match patients to clinical trials
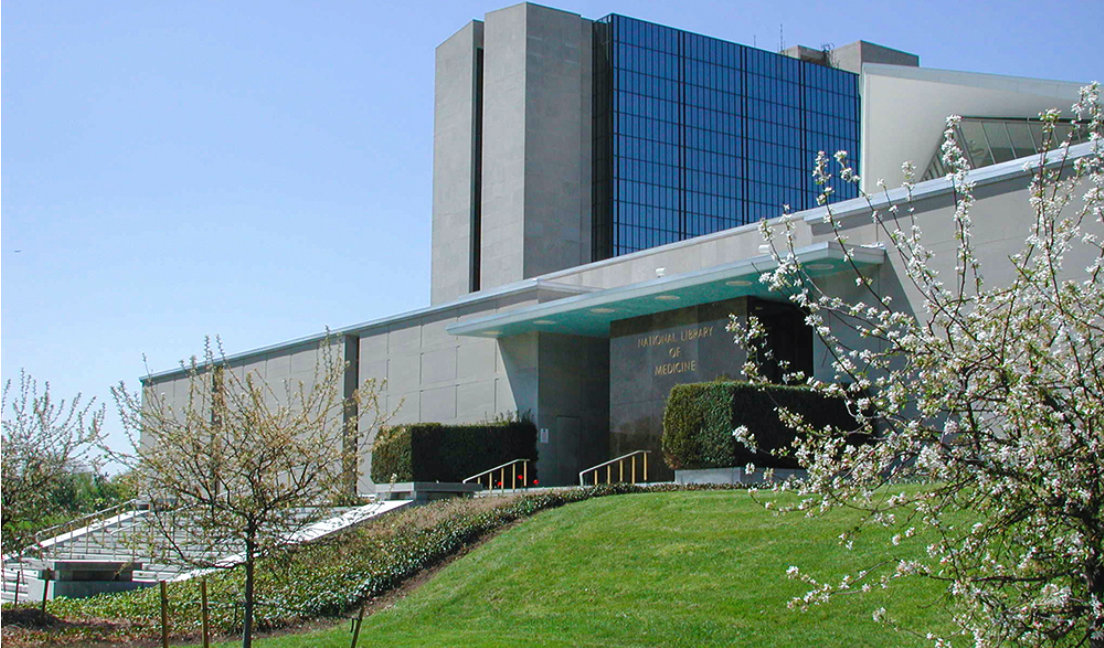
Few organizations in the world do more to turn biomedical and behavioral research into better health than the National Institutes of Health, its 27 institutes and centers and more than 18,000 employees.
One of those institutes is the National Library of Medicine (NLM). Considered the NIH’s data hub, NLM’s 200-plus databases and systems serve billions of user sessions every day. From PubMed, the premier biomedical literature database, to resources like Genome and ClinicalTrials.gov, NLM supports a diverse range of users, including researchers, clinicians, information professionals and the general public.
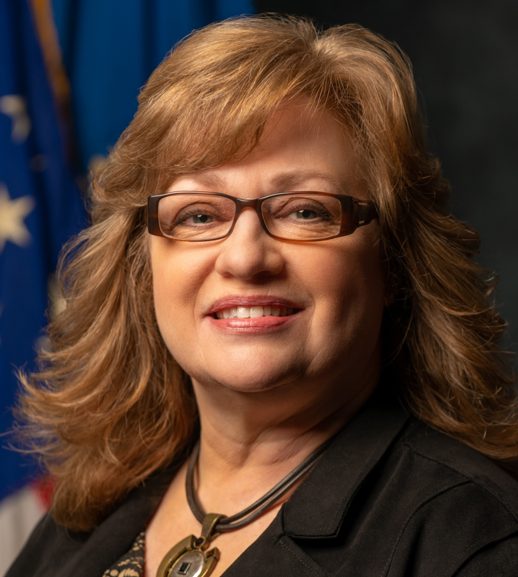
With so many users coming to its sites looking for a variety of information, NLM is always looking for new ways to enhance its products and services, according to Dianne Babski, Director of the User Services and Collection Division. NLM has been harnessing emerging technologies for many years but was quick to see how generative AI and large language models (LLMs) could potentially make its vast information resources more accessible to improve discovery.
Focus on innovation
“We’ve jumped into the GenAI [AI] arena ,” Babski said. “Luckily, we work in a very innovative institute, so staff were eager to play with these tools when they became accessible.” Through the Science and Technology Research Infrastructure for Discovery, Experimentation, and Sustainability (STRIDES) initiative, NIH researchers have access to leading cloud services and environments.
For her part, Babski is leading a six-month pilot project across NLM focused on 10 AI use cases using GenAI. The use cases are divided into five categories: product efficiency and usage, customer experience, data and code automation, workflow bias reduction, and research discovery.
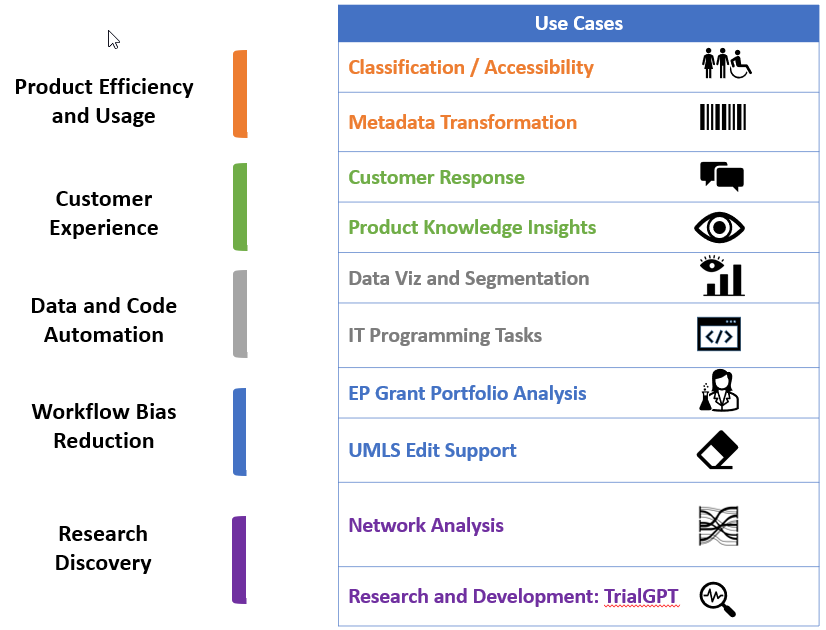
The participating cloud service providers gave NIH access to a “firewalled, safe environment to play in, we’re not in an open web environment,” Babski explained. As part of this pilot program, NLM is also providing feedback on the user interface that it’s been creating for one of the provider’s government enterprise system.
Reducing recruitment challenges in clinical trials
One use case with potentially significant implications focuses on the work in ClinicalTrials.gov. Researchers, clinicians and patients use this NLM database to search for information about clinical research studies worldwide.
While clinical trials are pivotal for advancing medical knowledge and improving patient care, one of the most significant challenges in conducting them is patient recruitment. Identifying suitable candidates who meet specific study criteria is a time-consuming and resource-intensive process for researchers and clinicians, which can hamper the progress of medical research and delay the development of potentially lifesaving treatments.
Recognizing the need to streamline clinical trial matching, NLM created a prototype called TrialGPT. Using an innovative LLM framework, TrialGPT is designed to predict three elements of patient eligibility for clinical trials based on several criteria. It does so by processing information from patient notes to generate detailed explanations of eligibility, which are then aggregated to recommend appropriate clinical trials for patients.
Early results have demonstrated TrialGPT can accurately explain patient-criterion relevance and effectively rank and exclude candidates from clinical trials. However, two challenges were also noted, according to an agency brief: the model’s lack of intrinsic medical knowledge and its limited capacity for medical reasoning.
To address these challenges, the NLM project team plans to augment LLMs with specialized medical knowledge bases and domain-specific tools.
Babski said implementing TrialGPT has the potential to deliver a more efficient and accurate method for matching patients to trials. “While currently only available as a research prototype, we see its potential as a great resource for clinicians to help find patient participants for these different types of trials,” she said.
Lessons learned
As NLM continues to pioneer and experiment with AI-driven use cases like TrialGPT, Babski said several vital recommendations and lessons have emerged. “One of the biggest things I’ve taken away from this is that it’s way more work and complicated than you think it’s going to be,” she said.
For instance, there is a steep learning curve for people to get comfortable with these new tools. But at the same time, that process also allows participants to develop new technical skills, such as running Python code and working in notebook environments.
Effective collaboration and interdisciplinary teamwork are also essential. According to Babski, the pilot program has been successful because NLM was able to not only assemble a “dream team” of domain experts, data scientists, and engineers but also established a community across NIH—currently more than 500 people strong—that is energized and motivated to share their work and support one another. “Everyone has a interesting use case and they are rolling up their sleeves, and trying to figure out how to work with GenAI to solve real work problems,” she said.
Babski also follows a checklist of goals to be applied to any Generative AI pilot:
- Experiment and develop best practices for LLMs in a safe (behind the firewall) “playground” environment.
- Create a proof of concept that applies to the agency’s work.
- Measure results to ensure utility and safety (e.g. NIST guidelines).
- Develop workforce skills in generative AI.
For other agencies and organizations looking to explore the potential of AI technologies, Babski shared that it’s essential to embrace a culture of adaptability. “You have to be OK with pivoting halfway through,” she said. “We were trying to do data visualization work, and we just realized that this isn’t the right environment for what we were attempting, so we pivoted the use case.”
Ultimately, NLM’s use cases, including TrialGPT, highlight the transformative impact of GenAI and cloud-based platforms on healthcare innovation. By leveraging these technologies, NLM is likely to improve future healthcare delivery and patient outcomes globally.
Editor’s note: This piece was written by Scoop News Group’s content strategy division.