- Sponsored
- Insights
How PNNL is leveraging AI and ML to enhance infrastructure resiliency
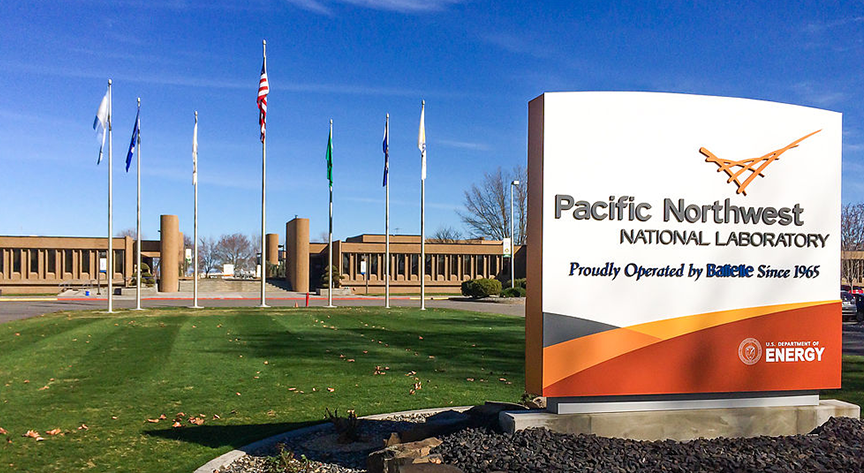
In recent years, the escalating frequency, intensity and cost of natural disasters have posed widespread challenges for federal, state and commercial officials. One such challenge is ensuring the resiliency of the nation’s infrastructure, including the energy grid.
That concern prompted scientists at the Department of Energy’s Pacific Northwest National Laboratory (PNNL) to begin exploring how artificial intelligence (AI) and machine learning (ML) could be used to predict wildfires and mitigate the impact of other potential natural disasters.
Pioneered by experts like Chief Scientist Andre Coleman, PNNL’s groundbreaking work has helped modernize disaster and emergency management by using AI and ML to make sense of vast amounts of satellite and geospatial data in near real-time. This helps public safety officials, utilities and land-use managers better prepare for pending disasters.
Where it started
According to Coleman, PNNL began experimenting in 2014 with a vision to harness imagery from satellite, aircraft and drones to swiftly assess hazards created by wildfires, floods, hurricanes and other weather-related disasters – and their impact on critical energy infrastructure.

“The original idea was to say, ‘Can we quickly make use of these sorts of imaging resources and assess a hazard? What’s the extent of the hazard? Where are we seeing the damage caused from the hazard?’” says Coleman. “And how do we relate those damages to critical energy infrastructure?”
To do this initial work, Coleman says PNNL built machine learning models and tools “to take that imagery and assess it in an automated way.” And then, over time, more machine learning algorithms were put into their models.
Where it stands
While PNNL’s focus was on near real-time response at the beginning, it evolved into proactive risk assessment that allowed emergency management and operations professionals to make decisions focused on mitigating risk well ahead of a disaster, such as vegetation management in and around critical infrastructure or replacing wood power poles with steels ones so they sustain through more extreme events.
This shift in focus was catalyzed by the increasing severity of wildfire events, especially historic fires that consumed more than 10 million acres in 2017 and again in 2020, prompting the recognition of wildfires as a national emergency.
“I think there was just this realization that fire is not the same as it once was,” says Coleman. “It burns more intensely; it moves faster.”
Advances in satellite technology have helped propel PNNL’s current approach, which involves integrating data from various satellite sensors, including passive and active sensors, to derive actionable insights. Passive sensors capture reflected energy, while active sensors penetrate cloud cover, storms and smoke, enabling continuous monitoring even in adverse conditions. By leveraging open-access satellite data and commercially collected imagery, as well as collaborating with agencies like NASA, the United States Geological Survey and the European Space Agency, PNNL can tap into a robust data ecosystem for its analysis.
In addition, the development of PNNL’s Rapid Analytics for Disaster Response (RADR) represents a significant milestone. The platform combines multi-modal imagery, AI and scalable cloud computing — thanks to partnerships with major cloud providers — with an infrastructure damage assessment tool. The tool helps users better understand the current impact and risk to infrastructure from wildfires, floods, hurricanes, earthquakes and more. Currently, once a provider collects and makes new imagery available – which typically takes about 4 to 6 hours – RADR can take that imagery, process and disseminate it in 7 to 10 minutes.
However, according to Coleman, newer satellite communications and increased automation are helping to drive this latency down to under an hour. In addition, this significant advancement in data collection and increase in the amount of data available is something that the RADR cloud platform has been planning for and is well-equipped to handle. For example, newer planned satellite sensors such as FireSat will provide a 20-minute satellite revisit, requiring a highly scalable system like RADR to generate analytics for these data.
In addition, PNNL’s work has caught the attention of a widening group of stakeholders, including the Department of Energy’s Office of Cybersecurity, Energy Security, and Emergency Response and the Joint Artificial Intelligence Center, which have played a crucial role in supporting PNNL’s endeavors. Collaboration with public and private utilities has further enhanced the research landscape, ensuring practical applications and alignment with industry needs.
Where it’s going
The complexity of wildfire detection and prediction necessitates continuous refinement of AI and ML algorithms. PNNL’s interdisciplinary team — comprising scientists, developers and cloud architects — remains dedicated to enhancing system capabilities. With the upcoming availability of specialized earth observation satellites, like NASA’s SWOT and NISAR, PNNL aims to expand its predictive capabilities further and extend its reach to a broader audience. “The idea is to keep adding and improving our algorithms, incorporate more sensors as they come online, continue to mature the capability and really work to get this out to as many end users as we can,” says Coleman.
PNNL’s efforts are also expanding beyond natural threats, to include a more holistic approach to safeguarding critical infrastructure, including the rise of physical as well as cyber attacks on critical infrastructure. By integrating environmental, cyber and physical security considerations, PNNL strives to build a more resilient energy grid capable of withstanding diverse challenges.
“A big role for us at the lab is to look at all these kinds of dimensions and say, ‘How do we protect the grid? How do we make it more resilient as our reliance on energy continues to increase?’’ says Coleman. “Through the various teams that are working on that, we have the opportunity to interface and consider how to move forward and build a better, more resilient system.”
As PNNL continues to innovate and collaborate, the future holds promise for a more resilient energy infrastructure. By leveraging the power of AI and ML, PNNL stands poised to address the evolving threats posed by natural disasters and enhance grid resiliency for generations to come.
The report was produced by Scoop News Group for FedScoop, as part of a series on innovation in government, underwritten by Microsoft Federal. To learn more about AI for government from Microsoft, sign up here to receive news and updates on how advanced AI can empower your organization.