IRS’s AI system to flag returns for audit may include unintended bias, report finds
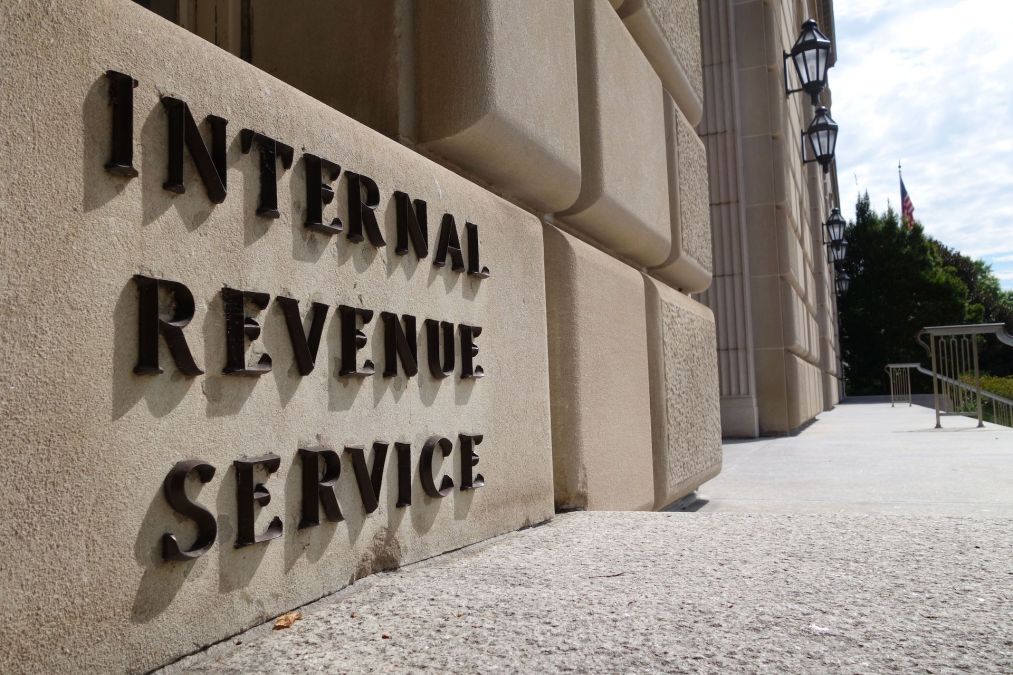
The IRS’s primary tool for flagging tax returns for audit is a “first-wave” AI system that includes inputs from humans, according to a new watchdog report, opening the door for unintended bias at a time when the agency is attempting to combat racial disparities in auditing.
The Government Accountability Office found no evidence that the tax agency has conducted a “comprehensive review of the rules and filters contained” in its Dependent Database, an automated program that identifies returns with possible noncompliance risk. The DDB is considered first-wave AI by the GAO due to it having “expert knowledge encoded into a computer system.”
“While IRS regularly reviews the program, the review process does not comprehensively consider data inputs and assumptions that could inform IRS about the demographic equity of the audit selection process, creating the potential for unintended bias in audit selection,” the report stated. “For example, GAO found that some risk scores contained in the DDB program vary by sex, which could skew selection, and have not been updated since 2001.”
A 2023 Stanford University study found that Black taxpayers are roughly three-to-five times more likely to be audited than filers of other races. The IRS later confirmed the study’s findings, with Commissioner Danny Werfel writing in a letter to Congress that the agency would be “laser-focused” on addressing racial disparities in auditing.
The GAO noted that the tax agency does not collect data about taxpayers’ race and ethnicity, meaning that predictions about a return’s risk for noncompliance with tax codes doesn’t take either factor into account. But according to the GAO, IRS research still shows “the existence of racial disparities in audits,” with “unintentional algorithmic biases” identified as a possible source.
“Specifically, that research noted (1) limitations in the data used to determine residency and relationship tests for [Earned Income Tax Credit] eligibility, and (2) outdated models as possible contributions to algorithmic bias and, consequently, racial disparities in audits,” the report states.
Once a return is flagged by the DDB program, it is then evaluated by the agency’s Systems Research and Application (SRA) model, which determines the filer’s risk score. Considered second-wave AI, the SRA is a data-mining and machine-learning model that the IRS uses to pinpoint audit patterns and predict outcomes.
The GAO identified “some components” of the IRS Wage & Investment Division’s “automated audit selection process that could potentially skew selection toward returns with certain demographic characteristics that may not necessarily represent returns with the highest risk of noncompliance.” The SRA ranks risk scores from highest to lowest, and W&I starts with the highest until meeting “its predetermined audit workload,” the watchdog noted.
The GAO pushed the IRS to abide by its AI accountability framework, particularly with regard to “a variety of monitoring activities” that should be followed “to ensure AI systems function as intended.”
“The agency may be missing opportunities to improve the likelihood that IRS is properly identifying returns at highest risk of noncompliance if it does not consider additional performance measures in reviewing its automated audit selection process,” the report said.
The GAO delivered six recommendations to the IRS regarding its audit selection processes, all of which were agreed to by the agency.