‘Morpheus’ ML model key to deciphering James Webb Space Telescope’s high-resolution images
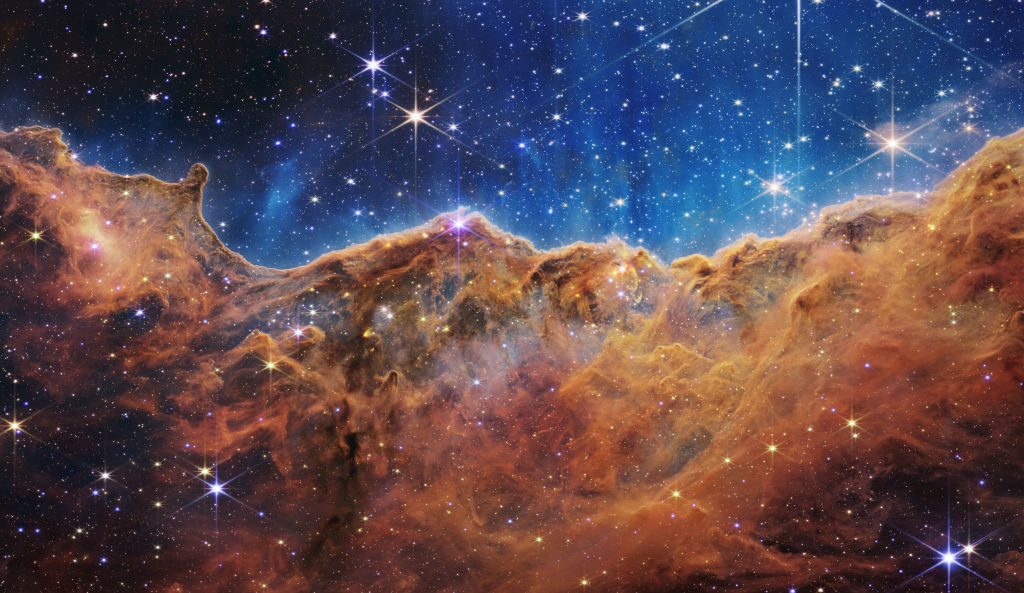
Scientists will continue to adapt the machine-learning model used to classify astronomical objects like stars and galaxies in the images taken by NASA’s James Webb Space Telescope to learn more about space.
The novel Morpheus model is a convolutional neural network that annotates every pixel of the full-color, high-resolution images of the distant universe the James Webb Space Telescope (JWST) captures.
In 2019, Ryan Hausen, now a research scientist at Johns Hopkins University, began writing the code for Morpheus — modifying a semantic segmentation algorithm used in computer vision to classify astronomical objects in Hubble Space Telescope data based on their shape. Morpheus was further trained, scaled and accelerated by the NVIDIA graphics processing unit-enabled (GPU-enabled) supercomputer, lux, at the University of California, Santa Cruz.
“From my perspective, things like James Webb and other upcoming telescopes are an exciting opportunity for computer scientists and people in machine learning to get their hands on novel, interesting data that is maybe outside the traditional computer vision realm,” Hausen told FedScoop. “And to do and work on things that benefit all of humanity.”
Hausen calls the supercomputer-enhanced model Big Morpheus, though it may be formally renamed when Brant Robertson, principal investigator on the project, eventually reports the model results.
Morpheus has already been adapted to classify sea ice when a telescope is pointed back at Earth and can handle images at different electromagnetic radiation spectrum wavelengths, as well as datasets for other tasks like detection
“You can use this type of semantic segmentation and scale it up, the way that we do with Morpheus, to apply it to different domains within science,” Hausen said.
Lux, which features NVIDIA V100 Tensor Core GPUs, is a key part of scaling. Funded with a $1.6 million grant from the National Science Foundation, the hardware was used to train and evaluate Morpheus’ ability to assign model probability that each pixel in a JWST image belongs to a spheroid, disk or irregular galaxy; compact stars; or background or sky classes.
GPUs accelerate Google’s TensorFlow library performing the computational heavy liftingL calculations involving lots of convolutions and linear algebra.
“We will be continuing to use lux over the next few years to perform [artificial intelligence, machine learning] analyses of JWST surveys, including all of the largest extragalactic programs that will be observed with JWST,” Robertson said.