NIST confirms that facial recognition doesn’t work equally well for all
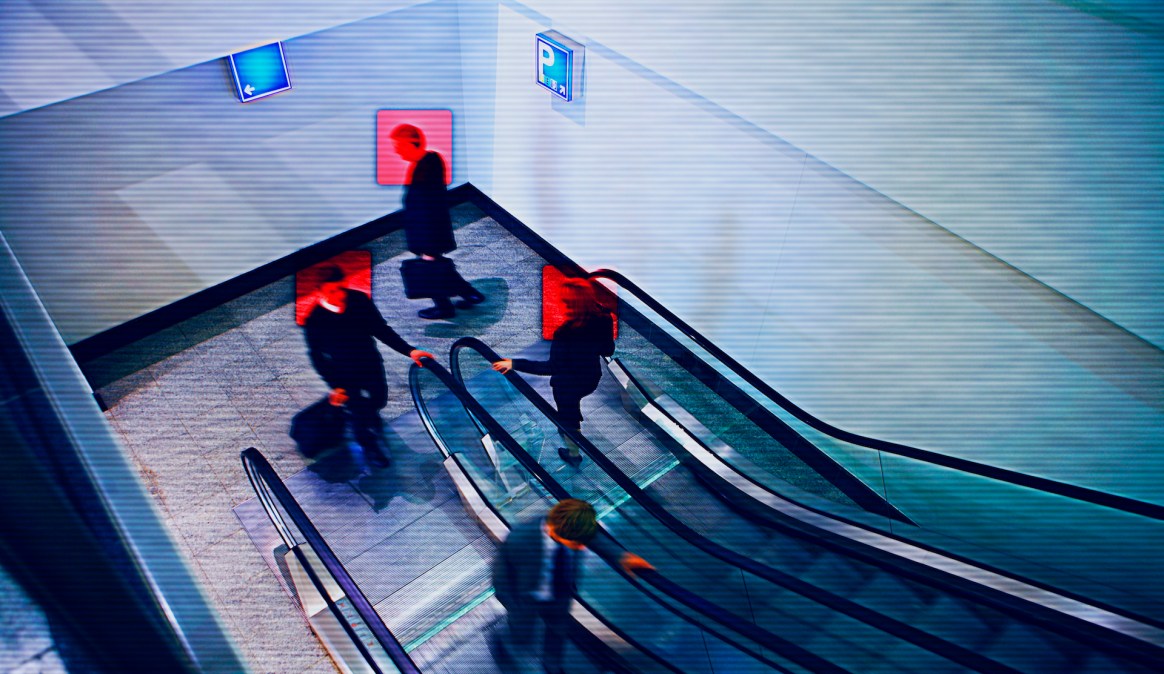
It’s a fact you’ve probably heard before: Facial recognition technology doesn’t work equally well for all people. In general, today’s facial recognition algorithms tend to be best at identifying white, male faces and can struggle when it comes to the faces of women or people of color.
Now, a new study from the National Institute of Standards and Technology (NIST) confirms this understanding.
“While it is usually incorrect to make statements across algorithms, we found empirical evidence for the existence of demographic differentials in the majority of the face recognition algorithms we studied,” Patrick Grother, a NIST computer scientist and the report’s primary author, said in a statement.
Translation: The algorithms misidentified people of color more than white people. They also misidentified women more than men.
The study, per usual for NIST’s work, is pretty robust. NIST evaluated 189 software algorithms from 99 developers — “a majority of the industry” — using federal government data sets containing roughly 18 million images of more than 8 million people.
The agency evaluated how well those algorithms perform in both one-to-one matches and one-to-many matches.
Some algorithms were more accurate than others, and NIST carefully notes that “different algorithms perform differently.” However, in the one-to-one matching scenario there were higher rates of false positives (where the software wrongly considered photos of two different people to show the same person) for Asian and African American faces as compared to Caucasian faces. Sometimes this effect was quite dramatic — depending on the algorithm, Asian and African American faces were sometimes misidentified 100 times more than their white counterparts.
The results also show more false positives for women than men and more false positives for the very young and very old when compared to middle-aged faces. In the one-to-many scenario, there were higher rates of false positives for African American women.
The study found some other interesting things too. For example, algorithms developed in Asian countries didn’t show the same “dramatic” false-positive results in one-to-one matching of Asian and Caucasian faces. This, NIST says, suggests how the diversity of training data (or lack thereof) impacts the resulting algorithm.
“These results are an encouraging sign that more diverse training data may produce more equitable outcomes, should it be possible for developers to use such data,” Grother said.
In general NIST presents its findings without much interpretation. But the implications could be important for the ongoing debate in Congress about what to do with this technology. Some jurisdictions, like San Francisco, have banned the use of the technology. And though there are some proposals, federal-level regulation remains elusive.
“While we do not explore what might cause these differentials, this data will be valuable to policymakers, developers and end-users in thinking about the limitations and appropriate use of these algorithms,” Grother said.
NIST’s last Facial Recognition Vendor Test examined overall algorithm accuracy for 127 vendor algorithms and found that the technology has undergone an “industrial revolution” in the past five years that’s made it far more accurate. In that test, certain algorithms were about 20 times better at searching databases and finding matches when compared to tests from 2010 and 2014.