- Sponsored
- Modernization
For AI success, start small and iterate
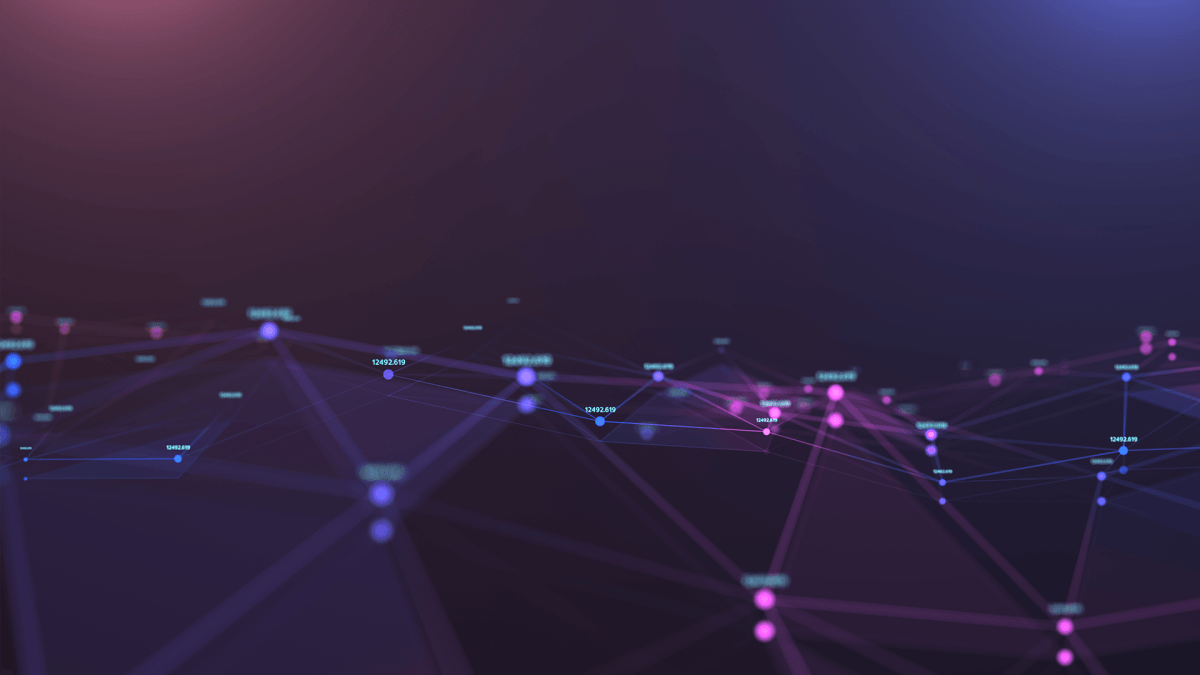
Artificial intelligence projects are most successful if they start small with specific use cases and grow over time, according to federal IT experts.
During a recent FedTalks panel on data, analytics and AI, experts from government and industry emphasized the importance of taking an iterative approach when developing AI applications.
Vimesh Patel, chief technology adviser of public sector for World Wide Technology, explained that AI use cases most often do best when agencies are “surgical” in choosing the purpose for the technology.
“What we generally like to do is sort of say, let’s identify those use cases, let’s map those to the kinds of analytics and techniques that would be best used to satisfy those use cases,” Patel said. “Let’s map that back to the kinds of data that you need. And then let’s map that to the technology that you need to underpin all that, you may run the risk of running into a few different silos, but I’ve seen that as the best way to get started.”
That’s how the Department of Agriculture is leveraging AI, said Chief Data Officer Ted Kaouk. For instance, the agency’s Agricultural Marketing Service used AI to improve the way it grades cotton in the United States. “We use equipment, machine learning and computer vision that reduces the level of manual work that’s required to grade that cotton and because we charge users for our services to grade that cotton, it reduces the cost to the end customer.”
But often, agencies take the opposite approach, Patel said.
They “will throw a lot of money at it, they’ll build out a big data lake, they’ll start buying all these technology platforms to hire a bunch of people,” he said. “And they’ll end up with like a data swamp without really any clear objectives for how they’re going to derive value out of it.”
Kaouk said USDA is working to take its small starts with AI and grow them into larger standards and platforms that can be used across the enterprise. “I don’t know if we have any one or two major lessons learned a regarding that, but I think rather little lessons that are learned iteratively along the way,” he said.
Patel agreed with this approach: “Start with very specific use cases. It’s OK if you end up in silos, because once you understand those use cases, you will know a lot more about what you need to scale and grow from there.”
View the panel discussion on Day 3 of the “Data, Analytics and AI” panel on FedScoop.
This article was produced by FedScoop and underwritten by World Wide Technology and Dell Technologies.