AI algorithms could be used to better forecast natural disasters, GAO report says
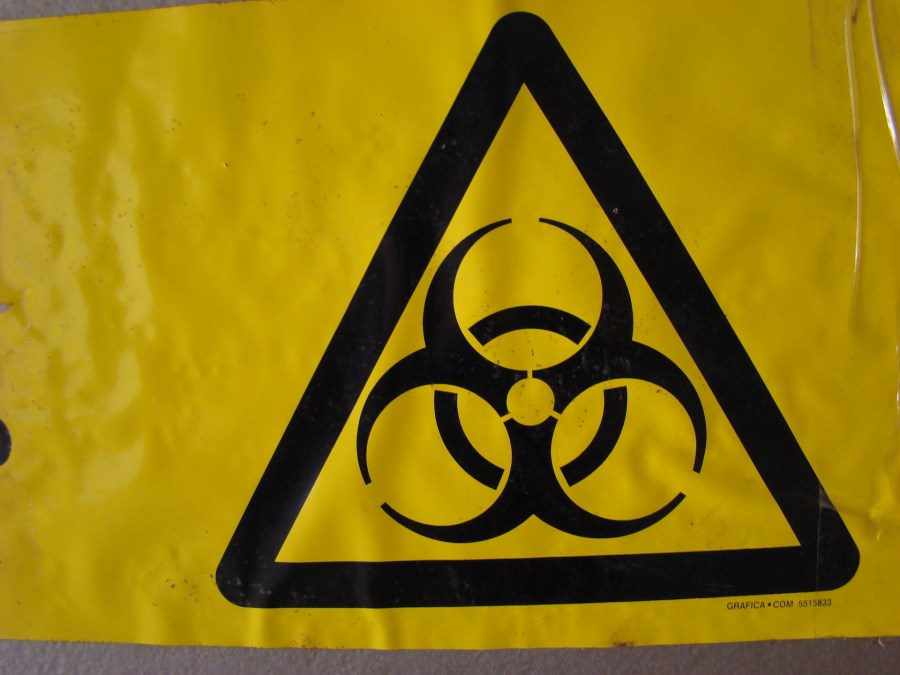
Artificial intelligence-driven algorithms can be used to better forecast models for natural disasters, saving lives and protecting property by rapidly analyzing massive data sets and identifying relevant patterns, a top government watchdog said in a report released Thursday.
Natural disasters result in hundreds of U.S. deaths and billions of dollars in damage annually, and machine learning AI tools could automate processes and glean new insights into weather patterns to improve warning time and preparedness during those events, the Government Accountability Office found.
“GAO found that machine learning, a type of artificial intelligence (AI) that uses algorithms to identify patterns in information, is being applied to forecasting models for natural hazards such as severe storms, hurricanes, floods, and wildfires, which can lead to natural disasters,” the GAO stated.
“A few machine learning models are used operationally — in routine forecasting — such as one that may improve the warning time for severe storms. Some uses of machine learning are considered close to operational, while others require years of development and testing.”
GAO conducted the study by reviewing the use of machine learning to model severe storms, hurricanes, floods and wildfires, in addition to interviewing government, industry, academia and professional organization stakeholders. The watchdog also reviewed key reports and scientific literature on the subject.
The GAO study found that applying machine learning to natural disaster detection could reduce the time required to make costly forecasts and increase model accuracy by more fully exploiting available data, using other data that traditional models cannot and creating synthetic data to fill gaps as well as reducing uncertainty in the forecasting models.
The GAO study also found some challenges with the use of machine learning and AI, such as: data limitations that hinder ML model training and result in lower accuracy in some regions, especially those in rural areas; concerns about bias and general distrust and misunderstanding of algorithms; the costliness of developing and running ML models; a lack of understanding of the data that is being modeled.
GAO highlighted five policy options that could mitigate those challenges: work toward better data collection, sharing, and use; create more education and training options; target hiring and retention hurdles and specific resource shortfalls; take steps to account for bias and build trust in data and ML models; and maintain current efforts.