Q&A: Director of NSF’s AI division discusses the tech’s limits and opportunities
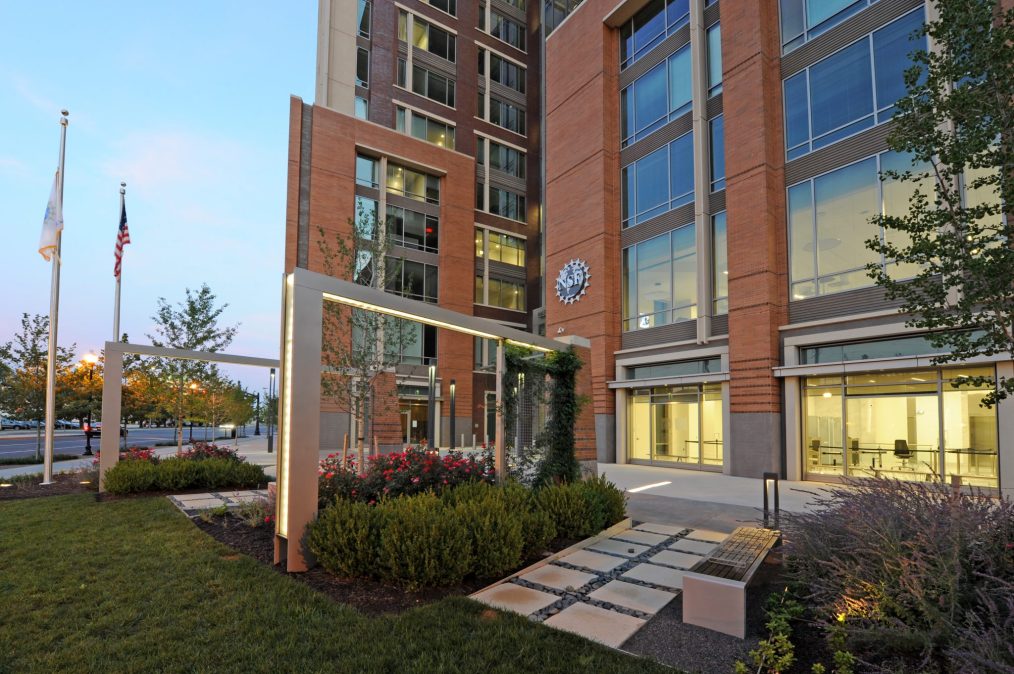
At the National Science Foundation, artificial intelligence is indisputably a major area of focus. The agency is funding new AI research centers, studying trustworthy AI systems, and even partnering with effective altruism-affiliated philanthropies on AI safety work.
There’s also an interagency group working developing plans for a pilot for a National AI Research Resource, which could, eventually, create an infrastructure for researchers across the country to do AI research and access data and compute resources. Meanwhile, the agency has another working group looking at the use of generative AI by reviewers — with new guidance expected in the next few months (In the meantime, reviewers are supposed to follow all existing rules on confidentiality and disclosure).
All this work comes as the agency continues to double down on research into the technology, which is quickly moving into the mainstream.
“We need to train people who are going to be creating the next cool AI algorithm that’s going to be useful in a zillion different settings,” Michael Littman, the division director for information and intelligent systems at the NSF, told FedScoop. “But we’re also interested in training the people who are going to take existing AI infrastructure and use it to solve a real-world problem that really matters.”
FedScoop recently sat down with Littman, who is also a computer science professor at Brown University, to discuss the state of artificial intelligence and NSF’s work on the technology. He explained that the agency is paying attention to emerging forms of AI, including generative AI systems, but also emphasized the limits of these kinds of systems.
“I’ve been reading some documentation from other governments where they’re saying, ‘Oh, yeah, this is going to help us solve the climate crisis,’” noted Littman. “And unless somebody has already written down ‘this is the solution of the climate crisis,’ these language models are not going to be able to articulate something that we haven’t already thought of.”
This interview has been edited for clarity and length.
FedScoop: What is your role at NSF and what you’ve been doing since you joined?
Michael Littman: I’ve been studying artificial intelligence for three decades — or something like that — but I’m on rotation with the National Science Foundation, where I’m serving as the division director for information and intelligence systems. The division that I head up is kind of the home base for AI and machine learning within the National Science Foundation. Even though … lots of the divisions are engaged in AI research in one way or another, this is really the division [where] that’s our main thing.
FS: What specific types of AI are you focusing on right now?
ML: We’re responsible for everything, so we have our fingers into everything. Both in terms of different styles of doing AI work, which include things like machine learning — which has had a huge impact in the last couple of years — but also more traditional knowledge-based and rule-based systems that have characterized the field for the decades before that.
One of the things that’s actually really important to a lot of the researchers in this community is: “How do we put the benefits of those two things together?” So machine learning systems are really terrific because you don’t have to have worked out as a human being exactly what all the details are. The system can kind of do that on its own, which is great. But it also means that we have less control over ultimately what the system does. We’re seeing that in things like the well-publicized chatbots where you can ask them questions and they will sometimes answer those questions reasonably and sometimes not.
The reason that they continue to have these weird behaviors is because there’s no obvious way to intervene to fix it. Because the systems are built using this machine-learning approach. We really would like to try to understand better: how do we get the benefits of both of these styles of doing AI?
FS: In your role as division director, how is generative AI coming up?
ML: I think what people in the community are interested in studying — and we’re encouraging them to do so — is addressing these questions of: Can we understand what they’re doing [and] how they’re doing it? And is there a way to make them more reliable, more trustworthy, in their behavior?
It’s amazing how fast these systems went from being kind of interesting curiosities that can kind of spew out rambly text to engaging in specific conversations and actually seeming to offer solutions to problems.
Personally, I’m very, very skeptical that the systems that we have now generate good solutions to problems that people haven’t already discussed. I think they’re really good at talking about the things they’ve seen on the internet, and to some extent, those are problems that people have solved.
I’ve been reading some documentation from other governments where they’re saying, “Oh, yeah, this is going to help us solve the climate crisis.” And unless somebody has already written down “this is the solution of the climate crisis,” these language models are not going to be able to articulate something that we haven’t already thought of.
What a lot of people in the field are pushing towards is using the language models as front ends, in a sense, as a kind of an interface that makes it easy for people to just express what it is that they’re trying to do. We’ve made sure at the NSF not to just swing the spotlight at language models and leave everything else in the dark. But all the other areas of AI that have been productive over the last couple of decades are still being supported.
FS: Are there areas of AI that aren’t getting enough attention?
We’re making sure that these other methodologies, more knowledge-based or more logic-based kinds of approaches, are still getting some support because we do think that ultimately the answer is going to involve a combination of these ideas.
FS: How do you see the role of an NSF in building national expertise in this? How do we increase the number of people who know how to use these systems?
ML: We need to train people who are going to be creating the next cool AI algorithm that’s going to be useful in a zillion different settings. But we’re also interested in training the people who are going to take existing AI infrastructure and use it to solve a real-world problem that really matters. And we’re interested in, at the K-12 level. How do we just make people aware of these kinds of systems, how they’re impacting their lives, and to be prepared for that future?