The Army looks to pave way for autonomous vehicles with new AI research
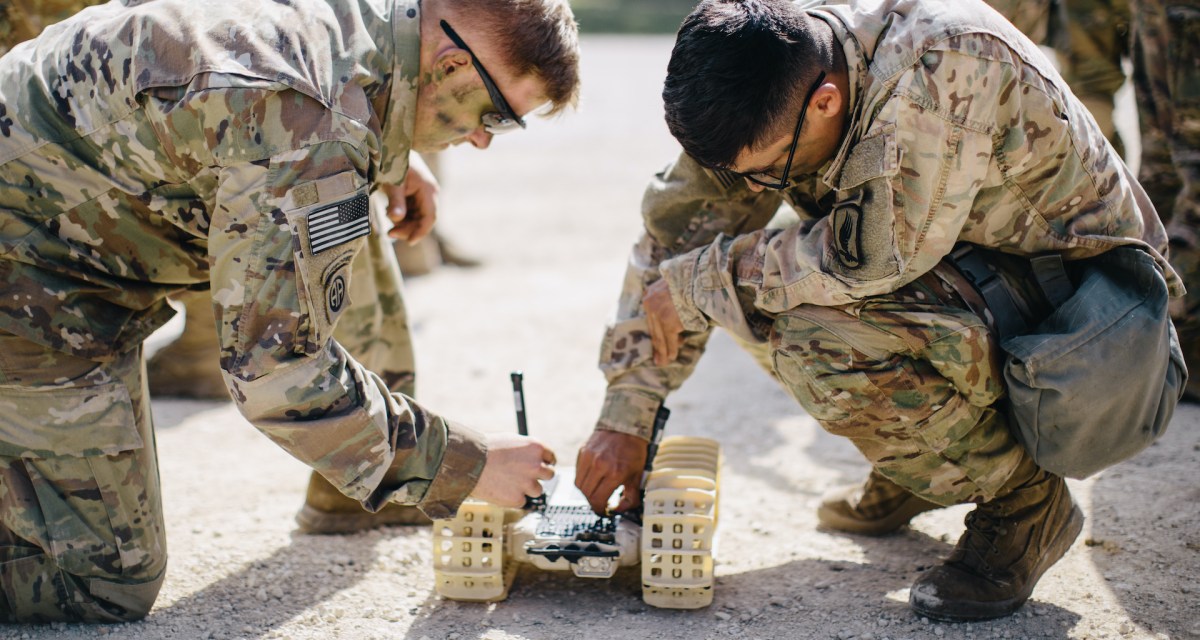
The Army has long wanted to put artificial intelligence in the driver’s seat of its ground vehicles, often with little success. But the service hopes new research could help it build more effective models for autonomous vehicles to learn how to steer through battlefields.
The new research, published in December at an AI conference, centers on the flavor of AI currently used in robots called “reinforcement learning.” The branch of machine learning emulates how humans learn: It takes an “agent” that uses prior knowledge gleaned from training datasets to make decisions in novel environments.
Members of the Army Research Lab worked with others at Google’s Deep Mind and Princeton University on the research. The Army, in a press release, said the research is “critical” to moving the Army closer to its “Multi-Domain Operations” future operating concept, one of its flagship modernization objectives to get different vehicles in different parts of a battlespace to work together.
“I am optimistic that reinforcement-learning equipped autonomous robots will be able to assist the warfighter in exploration, reconnaissance and risk assessment on the future battlefield,” said Alec Koppel, a research scientist at Army Research Lab and co-author on the recent paper.
In the past, the Army’s AI systems have not been able to navigate through unfamiliar territories, faltering when objects could not be easily classified. The new research attempts to advance a “gradient” method, where agents can maximize for many objectives ranging from object avoidance, speed or human safety. To decide how to accomplish objectives, the system uses what are called “policies” — a common word for military affairs, but in this case referring specifically to how an agent works through the possibilities of approaching new environments. It’s different than many current models that can only maximize for specific outputs that often conflict with how best to operate in battle.
The research is one part of a long journey that the Army has been on and will continue to work through. The Army has set a goal to have its ground vehicles be independent “teammates” to human-piloted systems, a prospect that could keep soldiers out of harm’s way and collect valuable data. But that reality hinges on the ability of its AI teammates to make adaptable decisions and find the best mix of using prior knowledge and diverging from the training data.
“To facilitate reinforcement learning for [Multi Domain Operations], training mechanisms must improve sample efficiency and reliability,” Koppel said, referring to how agents need to maximize an AI system’s ability to make policies for many outputs that are specific to battlefield operations.
Another benefit the Army is touting from its new research is a more efficient use of training data. Making datasets large enough for ground vehicles is expensive, the Army said in its release. The AI systems need massive vats of data to learn anything useful. But with the new gradient policy system, researchers hope that training data will, byte-for-byte, become more valuable and useful.
“These innovations are impactful to the U.S. Army through their enabling of reinforcement learning objectives beyond the standard cumulative return, such as risk sensitivity, safety constraints, exploration and divergence to a prior,” Koppel added.