DOJ official: Data analytics resulting in more efficient health care fraud detection
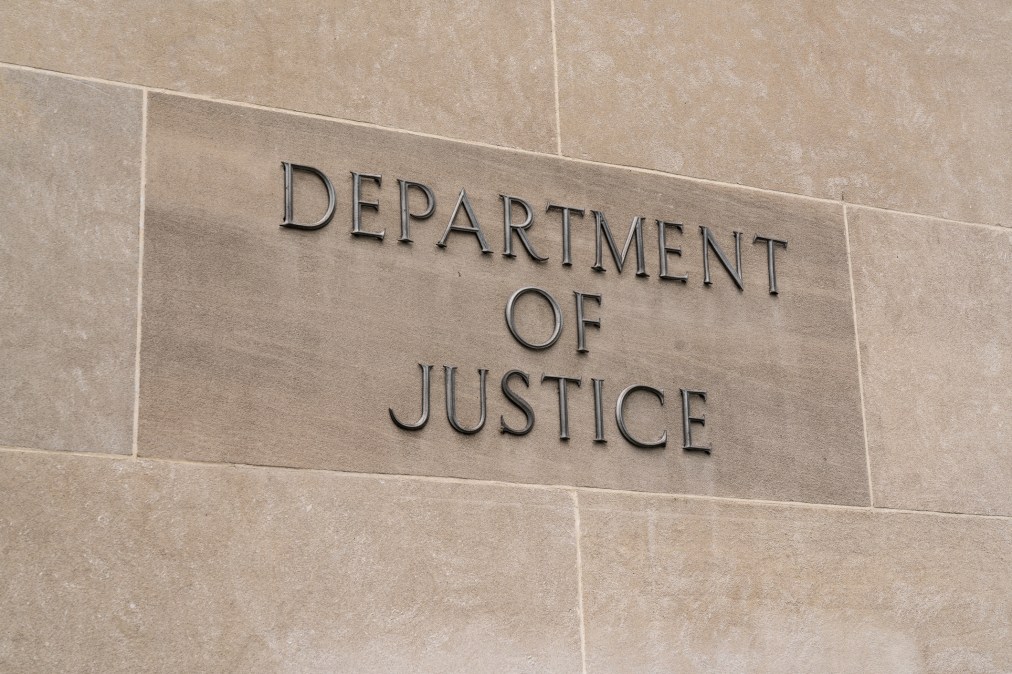
Data analytics and machine learning tools are driving health care fraud prosecutions led by law enforcement and federal health agencies, according to a senior Department of Justice official.
Jake Foster, acting principal assistant chief within the fraud section of the DOJ’s criminal division, said Wednesday at a Federal CIO Council event that analysis of aggregated data was providing previously unobtainable crucial insights.
While a legal case cannot rely on data alone, Foster said the ability to spot patterns in large datasets is resulting in the more efficient use of prosecutors’ resources by facilitating more targeted initial inquiries.
“These are the type of things that have been going on for years … that the old type of policing would not have caught,” he said. “The way that we do it [now] is through data analysis.”
The DOJ official cited an example of a case prosecuted last March, in which 16 Michigan and Ohio-area defendants, including 12 doctors, were sentenced to prison for a $250 million health care fraud scheme. According to Foster, the DOJ’s investigation progressed after data analysis produced an initial list of physicians who were among the most prolific prescribers of opioids, and a preliminary investigation identified connections between certain subjects.
In that case, patients submitted to expensive, unnecessary and sometimes painful back injections to receive opioid prescriptions. The doctors were compensated for the expensive injections and agreed to work only a few hours a week to “stay under the radar” of the Drug Enforcement Agency, according to the DOJ.
Foster added that the use of data analytics is key to ensuring that federal agencies working to combat health care fraud return the most amount of money to taxpayers.