- Sponsored
- Sponsored Content
For artificial intelligence, most of the work comes after launch
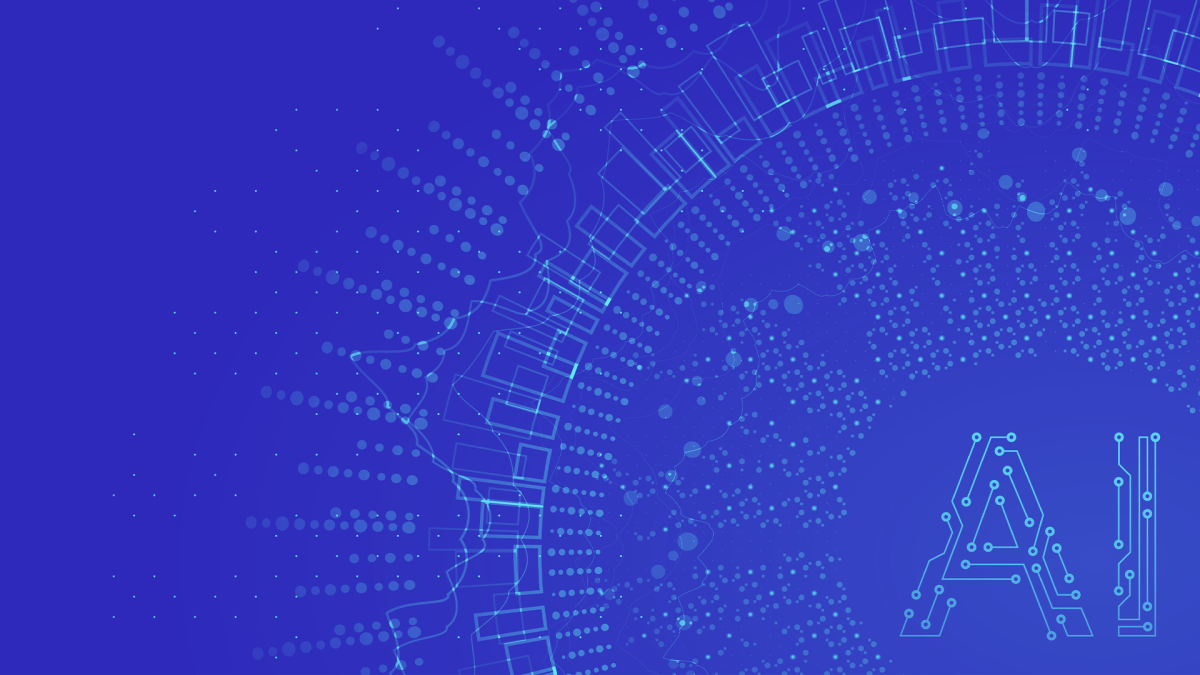
Successful artificial intelligence applications are difficult to build. But even more difficult than building them is the time spent after launch to manage and monitor a tool to make sure it is doing no harm.
This was a main thesis of Andrew Moore, vice president of engineering and general manager of cloud, artificial intelligence and industry solutions for Google Cloud, during his keynote at FedTalks 2020.
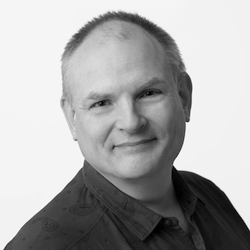
Andrew Moore, VP, Engineering & GM, Cloud AI & Industry Solutions, Google Cloud
Moore shared his lessons learned from building AI or machine learning applications to federal agency leaders at the virtual event, hosted by FedScoop on Nov. 17, 2020.
He explains that even though the team built a tool accurate enough to solve problems, after implementing it “in every single occasion, I ended up building a much larger team to do all the monitoring, improvement and ability to run new experiments for this machine learning system as it was carrying on through its life.”
For this reason, Moore said, when building AI applications, most of his time is spent “making sure that you’ve got something which is running securely, you’re able to understand and identify a problem if it starts to behave strangely, or if one of your customers is complaining that something really weird has happened.”
“When you’re about to run an AI system, or you’re in charge of an AI project, most of your thinking right from the start should be what you’re going to do after it’s been launched,” Moore said, stressing that the month of operationalizing the machine learning system afterwards is even more important.
AI for everyone
As AI capabilities becomes more advanced, the necessary skillsets to work with these applications has become less onerous and therefore more accessible for the existing workforce.
“I believe that now, we’re entering the third decade of the 21st century and we’re at the point where you do not need to be a Ph.D. expert in artificial intelligence in order to build AI,” he said. “We’ve just hit this threshold where regular folks, people who can play with spreadsheets or can modify Minecraft, that’s the level of technology skill you need in order to be able to build an AI.”
An auto ML system essentially replaces all of the messy, manual work involved in being a data scientist and democratizes it for the people who aren’t skilled in data science.
“And the idea is that here, someone who’s doing an important thing in an organization who needs to train up a system to do something should be able to if they can’t afford or they don’t have access to their own data scientists — they should be able to build stuff themselves,” Moore said.
View the full video of the keynote, “The Four Factors: How to Lead an AI Enterprise,” from Day 1 of FedTalks.
This article was produced by FedScoop and underwritten by Google Cloud.