Oversight questions loom over federal AI efforts
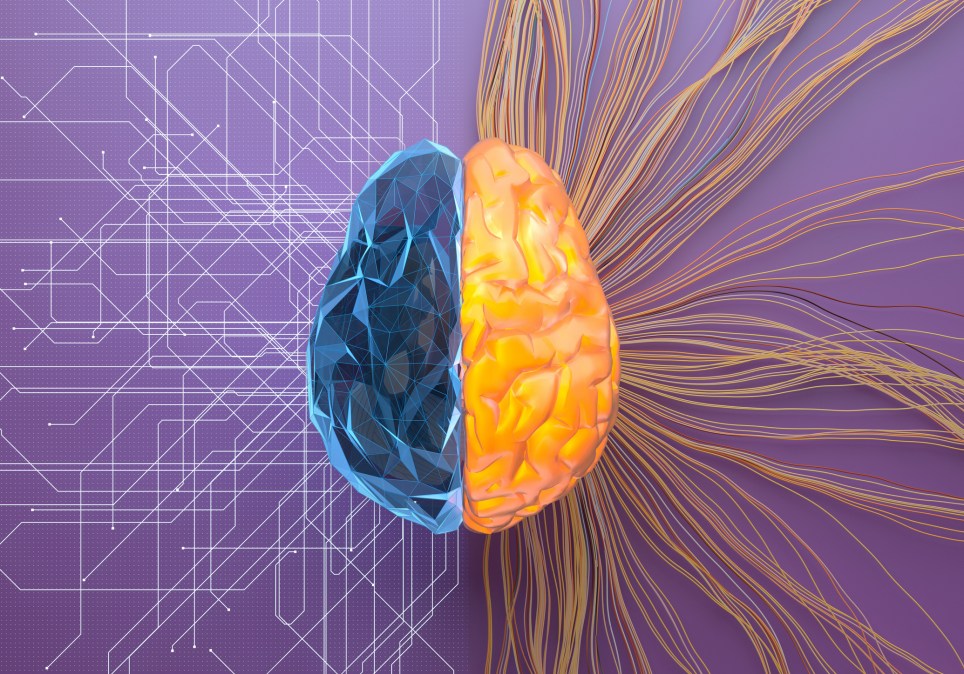
The Biden administration established several artificial intelligence bodies in 2021 likely to impact how agencies use the technology moving forward, but oversight mechanisms are lacking, experts say.
Bills mandating greater accountability around AI haven’t gained traction because the U.S. lacks comprehensive privacy legislation, like the European Union’s General Data Protection Regulation, which would serve as a foundation for regulating algorithmic systems, according to an Open Technology Institute brief published in November.
Still the White House launched the National AI Research Resource (NAIRR) Task Force and the National AI Advisory Committee, both authorized by the National AI Initiative Act of 2020, hoping to strengthen the U.S.’s competitive position globally, which may prove a losing battle absent oversight.
“Right now most advocates and experts in the space are really looking to the EU as the place that’s laying the groundwork for these kinds of issues,” Spandana Singh, policy analyst at OTI, told FedScoop. “And the U.S. is kind of lagging behind because it hasn’t been able to identify a more consolidated approach.”
Instead lawmakers propose myriad bills addressing aspects of privacy, transparency, impact assessments, intermediary liability, or a combination in a fragmented approach year after year. The EU has only the Digital Services Act, requiring transparency around algorithmic content curation, and the AI Act, providing a risk-based framework for determining if a particular AI system is “high risk.”
OTI holds foundational privacy legislation needs strong protections, data practices safeguarding civil rights, requirements that governments enforce privacy rights, and redress for violations. The White House Office of Science and Technology Policy is in the early stages of crafting an AI Bill of Rights, but that’s just one component.
The bulk of legislative efforts to hold platforms accountable for their algorithms has been around repealing Section 230 of the Communications Decency Act, which grants intermediary liability protections to social media companies, website operators and other web hosts of user-generated content. Companies like Facebook can’t be held liable for illegal content, allowing for a free and open internet but also misuse, in the case of discriminatory ads, Singh said.
Repeal of Section 230 could lead to overbroad censorship. OTI hasn’t taken an official position on the matter, but it does advocate for including algorithm audits and impact assessments in the federal procurement process to root out harm throughout the AI life cycle.
Currently there’s no federal guidance on how such evaluations should be conducted and by whom, but officials are beginning to call for more.
“Artificial intelligence will amplify disparities between people and the way they receive services, unless these core issues of ethics and bias and transparency and accessibility are addressed early and often,” said Anil Chaudhry, director of federal AI implementations at the General Services Administration, during an AFFIRM webinar earlier this month.
The Department of Justice’s Civil Rights Division has started identifying AI enforcement opportunities across employment, housing, credit, public benefits, education, disability, voting, and criminal justice. The division is also involved in multiple agencies’ efforts to create ethical AI frameworks and guidelines.
Legislation will take longer.
“We are studying whether policy or legislative solutions may also offer effective approaches for addressing AI’s potential for discrimination,” said Kristen Clarke, assistant attorney general, in a speech to the National Telecommunications and Information Administration on Dec. 14. “We are also reviewing whether guidance on algorithmic fairness and the use of AI may be necessary and effective.”
The work continues
The NAIRR Task Force continues to develop recommendations to Congress around the feasibility, implementation and sustainability of a national AI research resource, irrespective of the uncertainty surrounding federal oversight.
Members discussed NAIRR governance models at their October meeting and the data resources, testbeds, testing resources and user tools that could comprise it during their Dec. 13 session.
Some research groups contend investing in a shared computing and data infrastructure would subsidize Big Tech suppliers without implementing ethics and accountability controls, but tech giants Google and IBM pushed back that a federated, multi-cloud model would democratize researchers’ access to their AI capabilities.
“A big part of this is to provide, really democratize and make sure you have broad access to this resource for all researchers,” Manish Parashar, director of the Office of Advanced Cyberinfrastructure at the National Science Foundation and task force co-chair, told FedScoop. “And especially researchers that are part of underrepresented groups, as well as small businesses funded by the Small Business Innovation Research and Small Business Technology Transfer programs.”
The task force invited a panel of outside experts to its Dec. 13 meeting to share perspectives on how the NAIRR can account for privacy and civil rights to ensure trustworthy AI research and development. Speakers recommended building inclusive datasets and conducting impact assessments, as well as choosing a governance approach that treats equity as a core design principle.
Members are considering models like the ones NSF uses for its Extreme Science and Engineering Discovery Environment (XSEDE), Open Science Grid, Partnerships for Advanced Computational Infrastructure, COVID-19 High-Performance Computing Consortium, and federally funded research and development centers (FFRDCs).
“At this point it’s possible that maybe the existing models don’t meet the requirements,” Parashar said. “And we may want to think of some new or hybrid model that needs to be developed here.”
During the most recent meeting, Task Force Co-Chair Lynne Parker suggested the governance body grant NAIRR access to researchers approved to receive federal funds and process proposals from those who aren’t and private sector users.
The task force is leaning toward recommending contracting with a company to design and maintain the NAIRR portal, with the caveat that the resource should curate training materials for researchers of different skill levels.
A range of testbeds exist that could be included in the NAIRR, each with their own pros and cons in combating issues like bias.
For example, open-book modeling allows researchers to see if their algorithms perform better than a public test dataset, but absent lots of data there’s a danger an algorithm will only perform well in that situation.
Meanwhile closed-book modeling lets researchers design an algorithm with a training dataset before receiving the test dataset, but there’s no guarantee algorithms will perform significantly better.
Both models are also focused on machine learning predictive performance, when artificial intelligence also includes self-improving features, said Andrew Moore, director of Google Cloud AI and task force member.
Simulation testbeds allow for the end-to-end monitoring of AI system performance, sometimes across thousands of runs, but just one costs millions of dollars to create. The task force is leaning toward not recommending them.
Ultimately NAIRR can prevent agencies from duplicating each other’s AI testbed efforts.
“We do believe that we should provide access to testbeds,” Moore said. “Testbeds have unambiguously accelerated research in the past.”